Jeff Schueler’s Biography
Jeff Schueler is an experimental particle physicist specializing in improving particle detector performance through novel machine learning techniques. Born and raised in the Seattle area, he earned his Bachelor’s degrees in Mathematics and Physics at the University of Washington.
After graduating, Jeff served as a New York City Teaching Fellow at Maspeth High School in Queens, NY. As the school’s first physics teacher, he built up the school’s physics laboratory and curriculum from the ground up, introducing AP Physics C in his second year and mentoring students in critical problem solving and scientific reasoning.
Jeff then moved to Honolulu to pursue graduate studies at the University of Hawaiʻi at Mānoa, where he worked under Dr. Sven Vahsen investigating beam-induced neutron backgrounds at the Belle II experiment and worked on gas Time Projection Chamber (TPC) research and development. In his third year, he lived onsite at KEK in Tsukuba, Japan, and led the commissioning efforts of systems of shoebox-sized TPCs that remain in use to this day.
In the final year of his PhD, Jeff spearheaded the application of deep learning to enhance particle identification and directional reconstruction in gas TPCs. He developed a custom ResNet architecture that demonstrated, for the first time, vector-directional head/tail reconstruction of sub-10 keV nuclear recoils on real data—an important milestone toward competitive directional dark matter detectors.
Jeff received his PhD in 2022 and joined the University of New Mexico in early 2023 as a postdoctoral researcher in Dr. Dinesh Loomba’s research group, working on the MIGDAL experiment. The MIGDAL experiment is a rare event search experiment which aims to observe and characterize the Migdal effect in nuclear scattering for the first time. Early in his tenure, Jeff conceived the idea of applying object detection as a way to employ machine learning training on real data for rare event search experiments where the rare event signal is a composite composed of commonly observed species. He has since successfully demonstrated this idea in the MIGDAL experiment through the development of an end-to-end YOLOv8-based pipeline that he trained on real data to automate the experiment’s search for the Migdal effect. This work and its accompanying software are published in PRD.
His current research interests are generally related to narrowing sim-to-real gaps in particle physics experiments. This includes implementing techniques for data-driven training like those he introduced to MIGDAL, improving detector simulations with data-driven generative AI, and label-free inference. He is also interested in leveraging ML at the front end for rare event search experiments.
In his spare time, Jeff enjoys crossword puzzles, running, reading, boardgames, PC building, retro video games, cooking, hiking, and rock climbing. He is also a certified hydro homie!
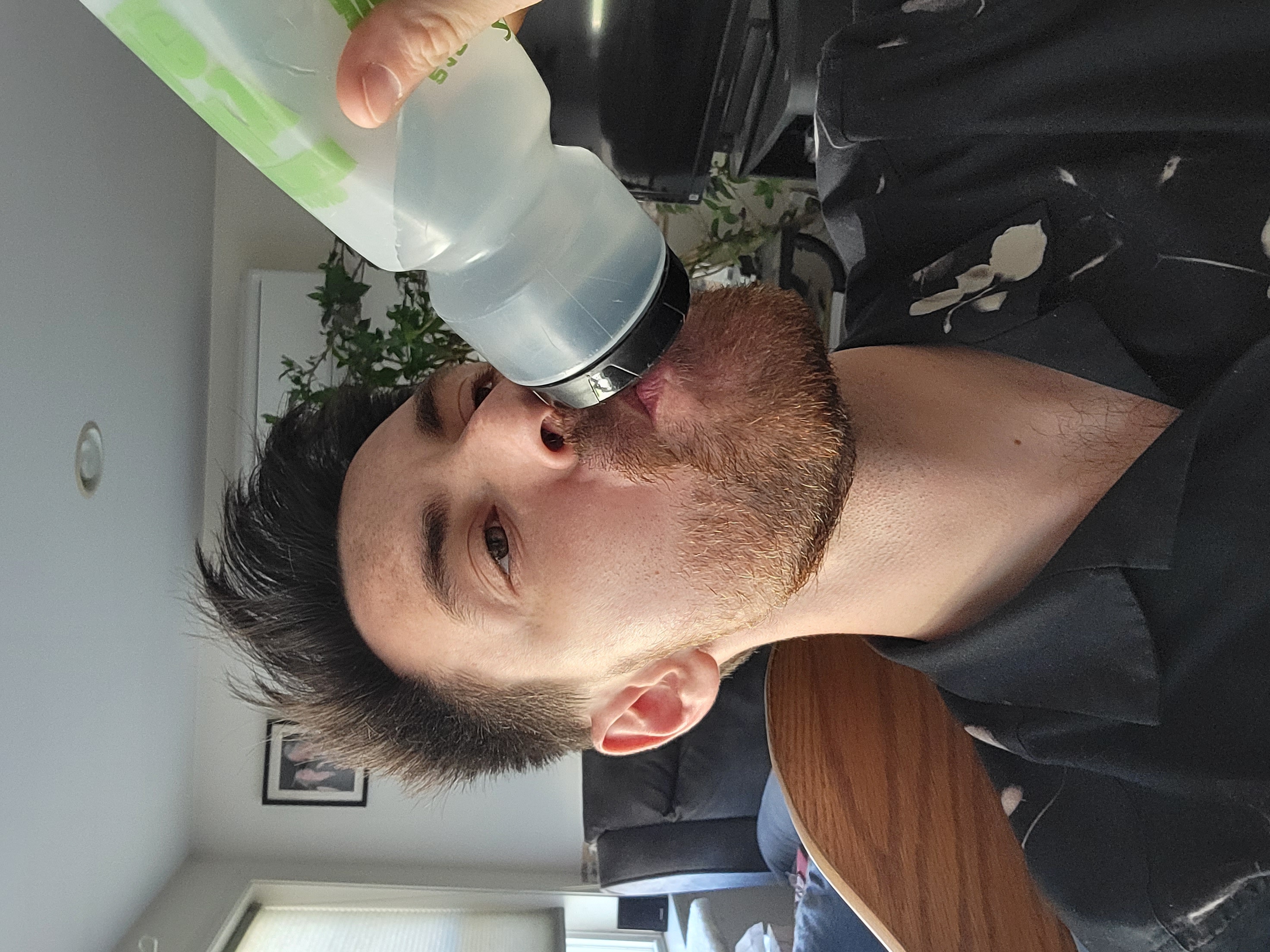
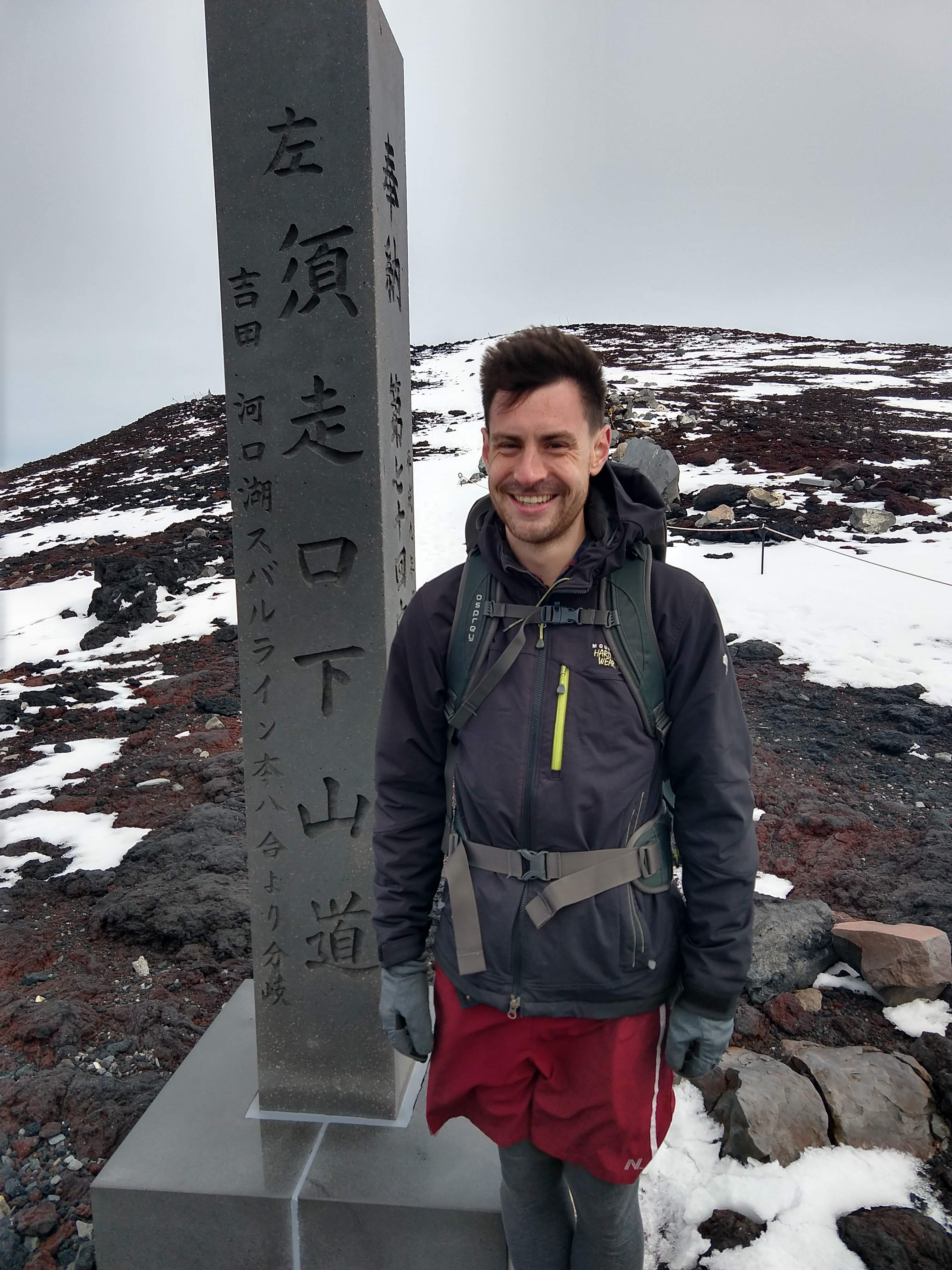